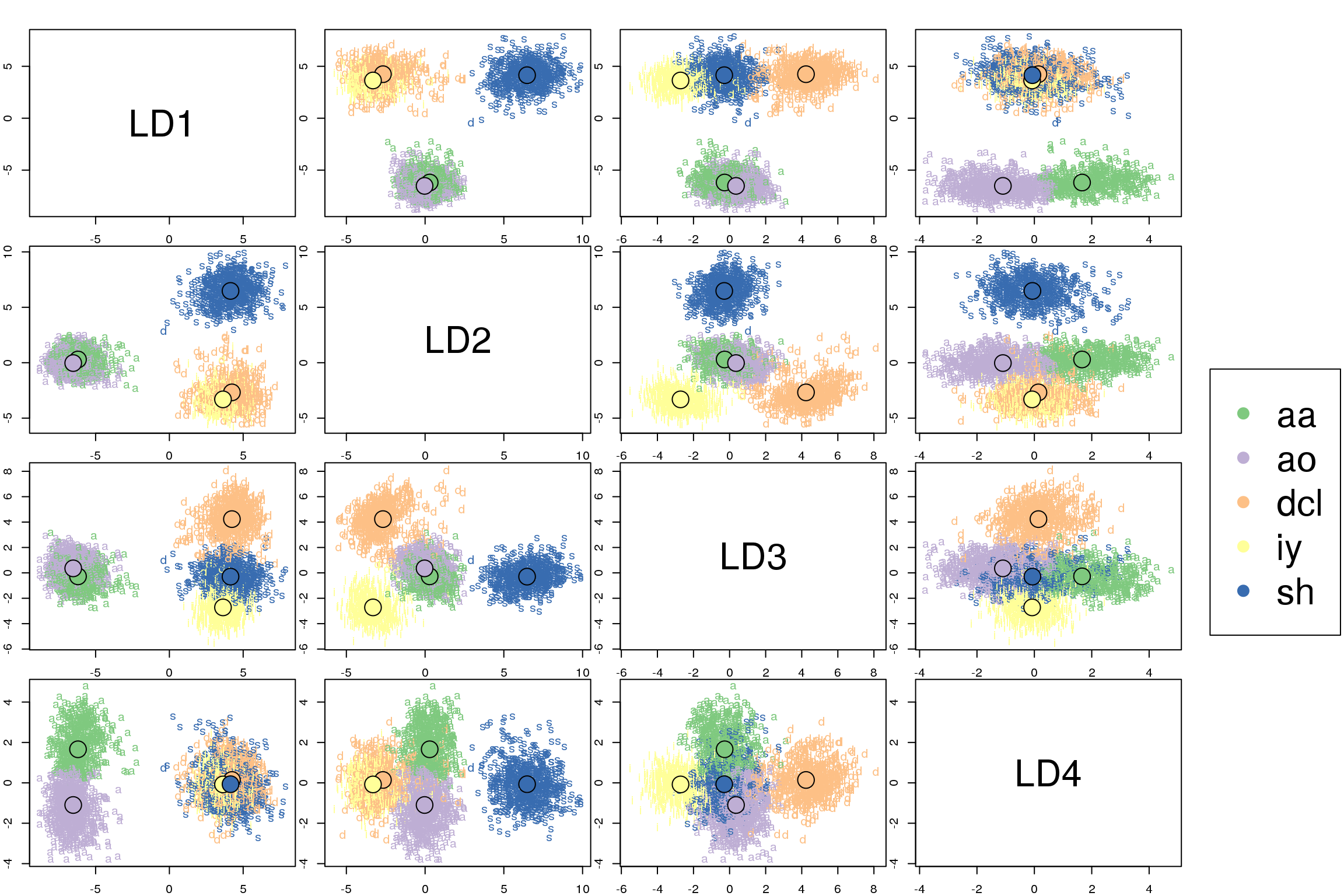
Data Visualization
Humans are visual creatures. Thus, visualization is one of the most important tools for conveying information and data scientists should be adapt at selecting appropriate visualizations.
Which plot is appropriate?
Choosing an appropriate plot for a given set of data can be hard because there are so many types of plots such as scatter plots, box plots, and histograms. Fortunately, I have created an overview of the most important plots, when they are appropriate, and how they can be used in R.
Posts about data visualization
The following posts deal with topics from data visualization.
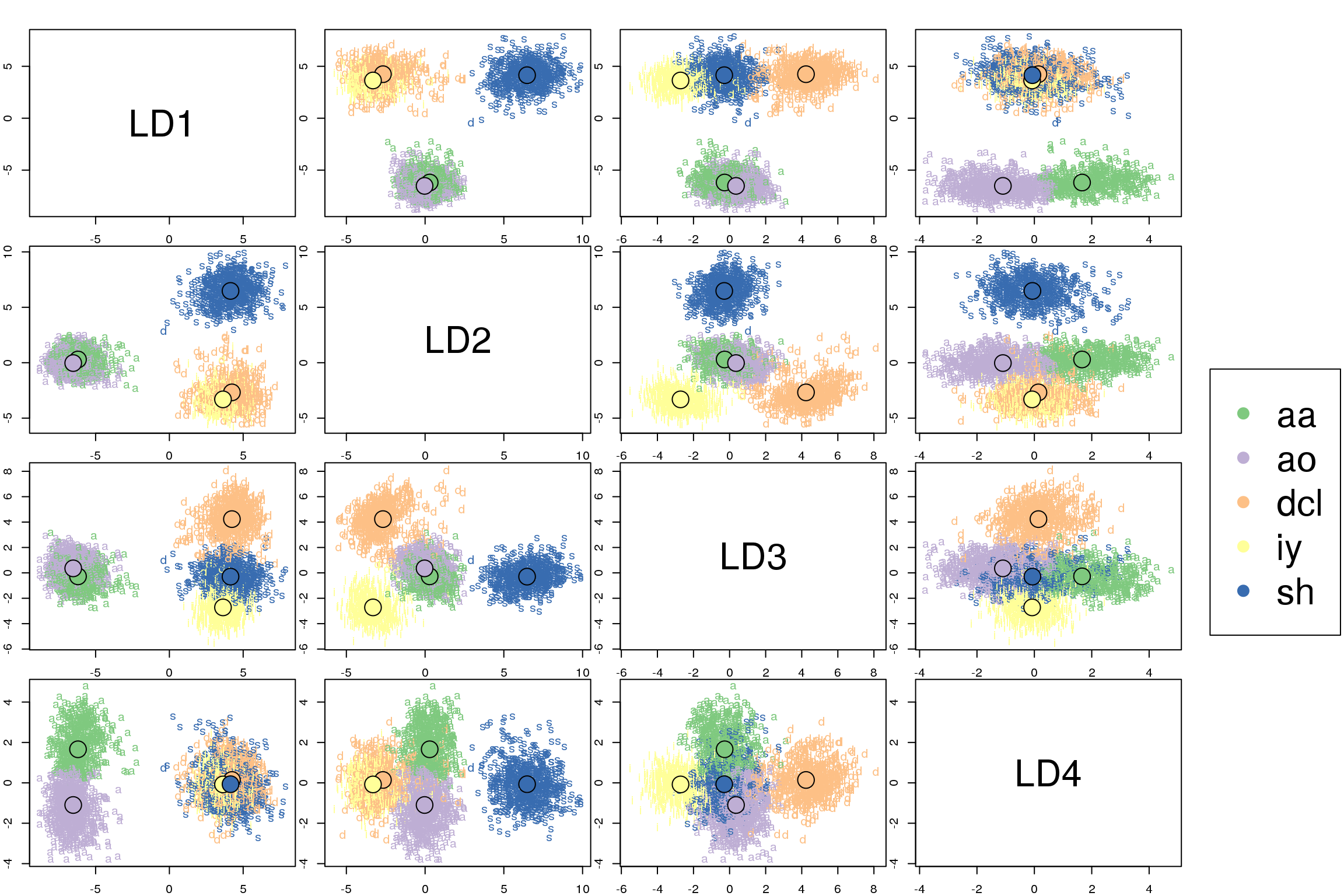
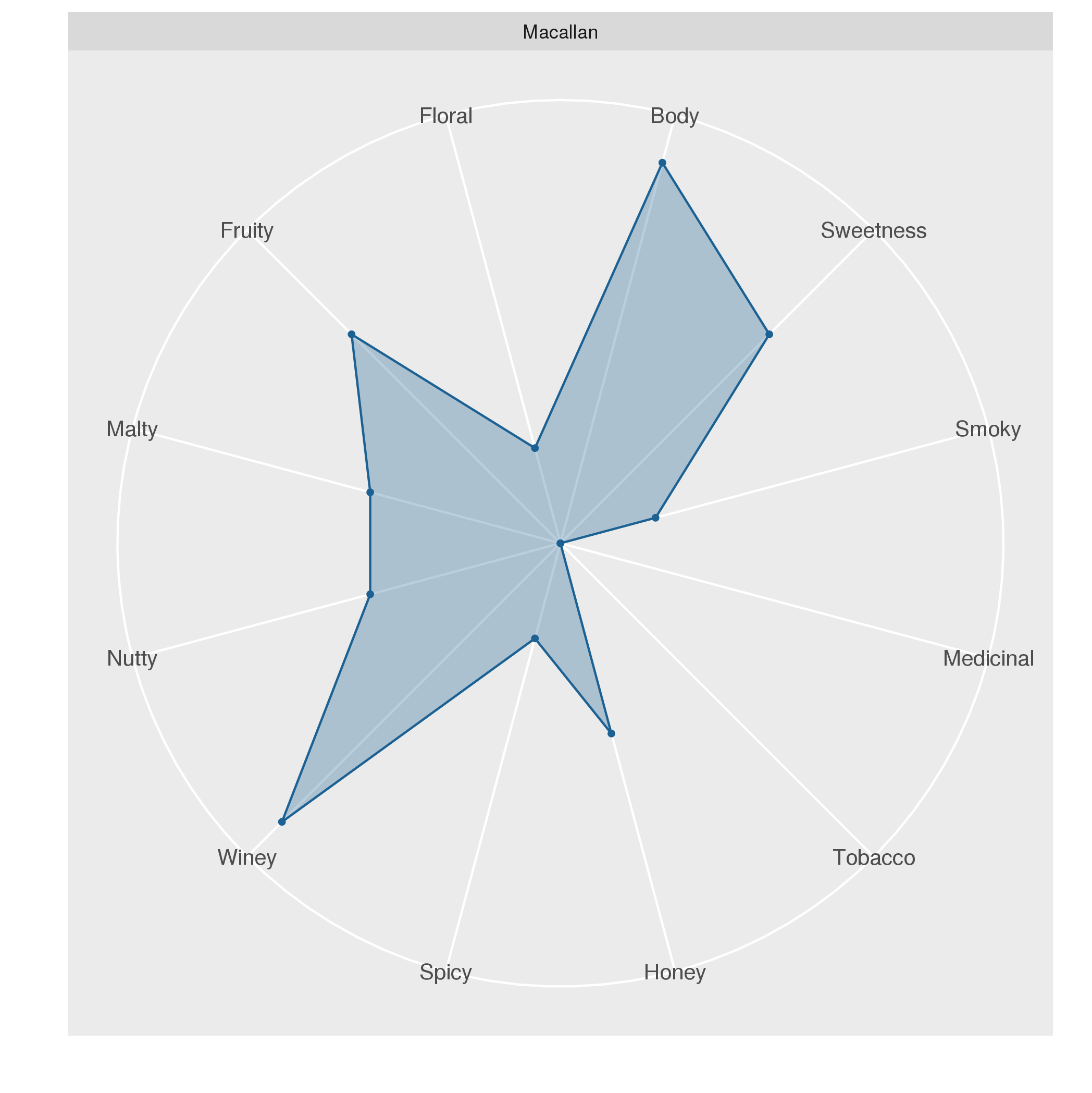
Radar plots
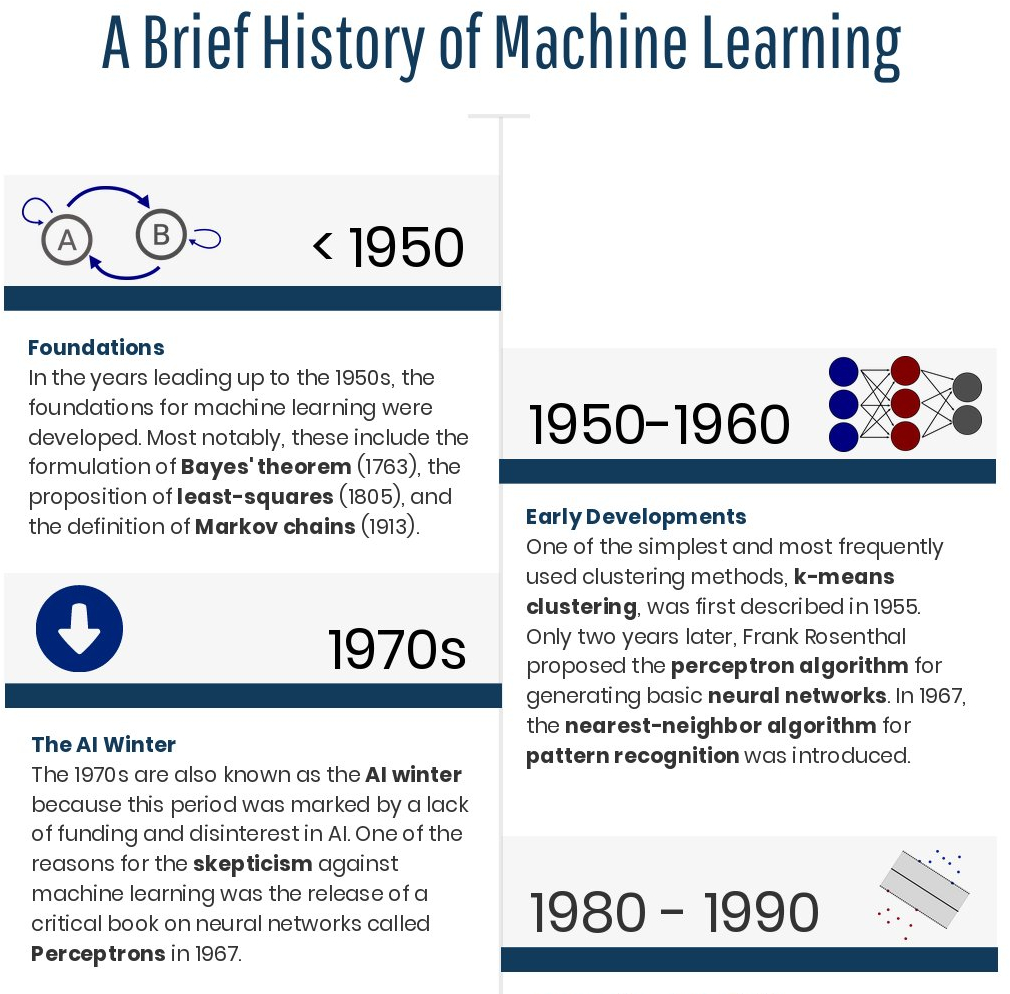
Getting Your Point Across with Infographics
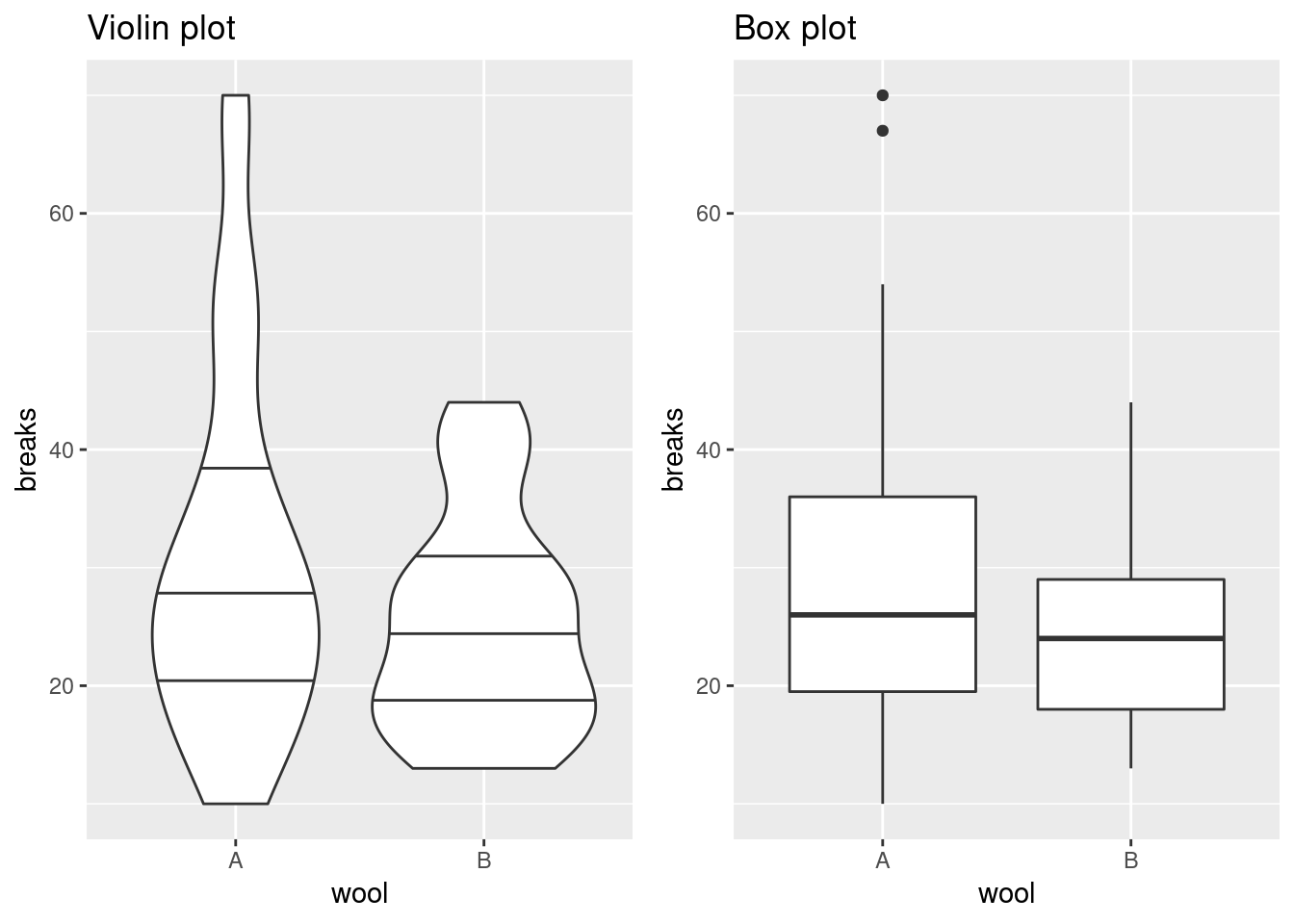
Box Plot Alternatives: Beeswarm and Violin Plots
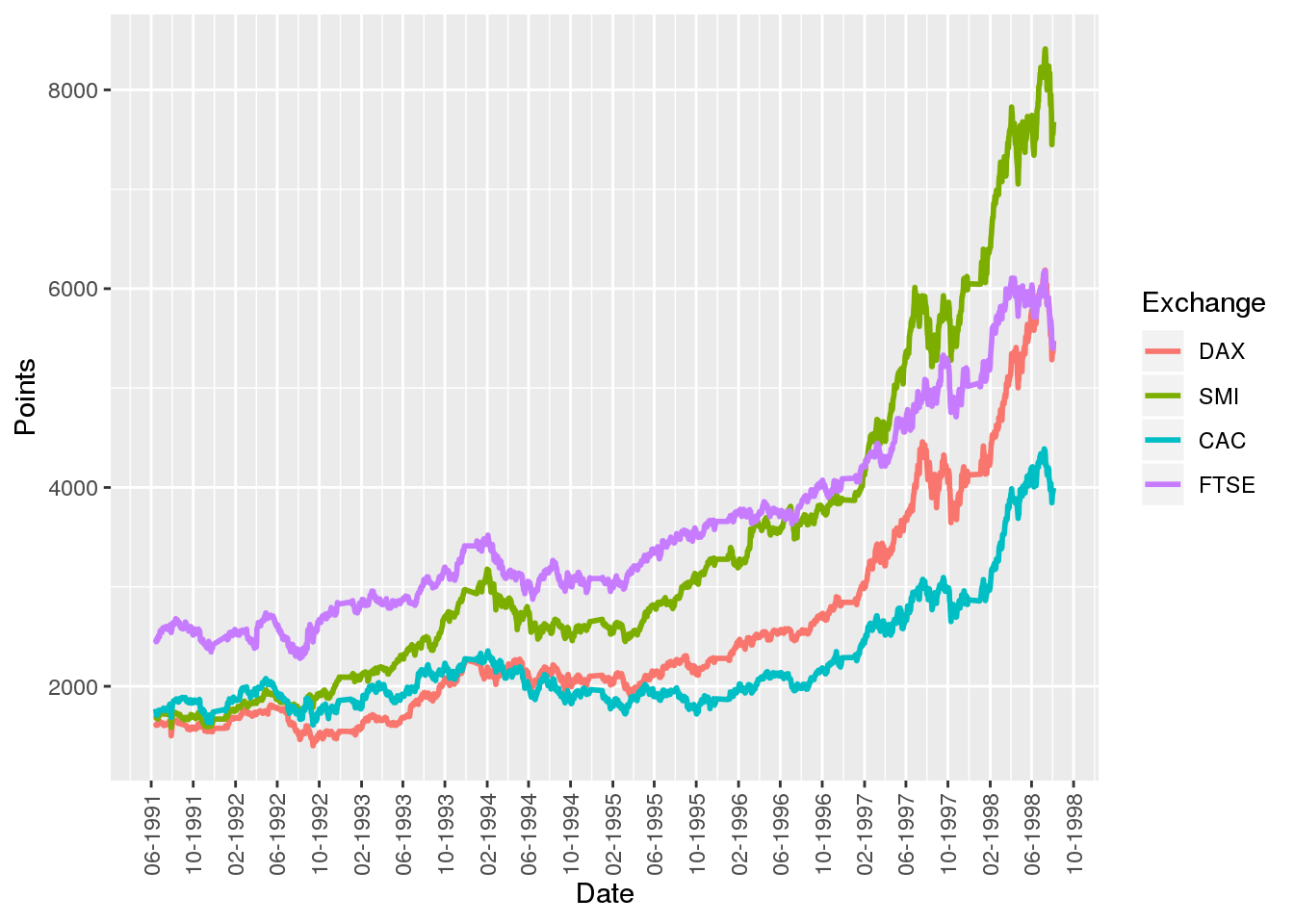
Visualizing Time-Series Data with Line Plots
The line plot is the go-to plot for visualizing time-series data (i.e. measurements for several points in time) as it allows for showing trends along time. Here, we’ll use stock market data to show how line plots can be created using native R, the MTS package, and ggplot.
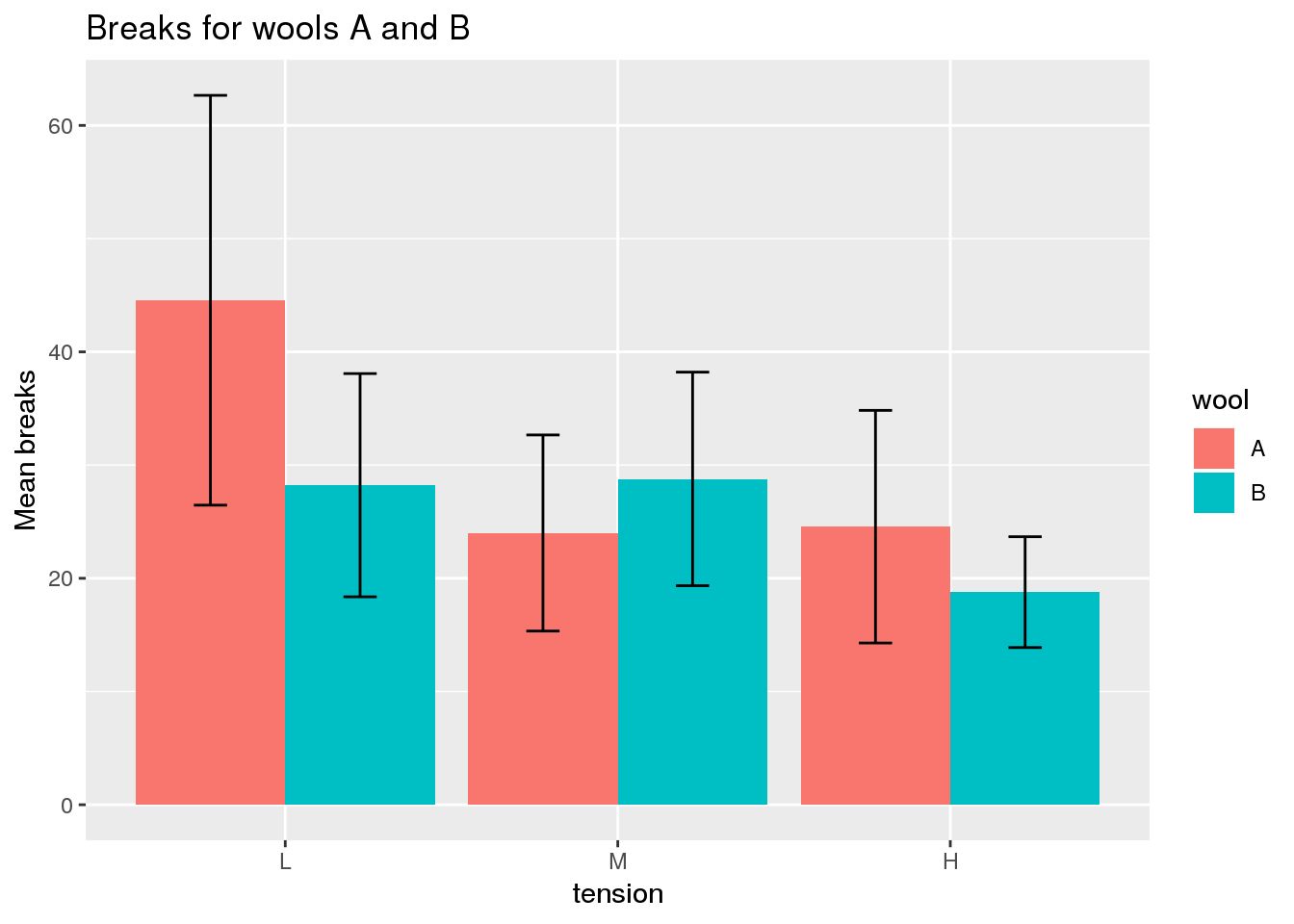
Bar Plots and Error Bars
Bar plots display quantities according to the height of bars. Since standard bar plots do not indicate the level of variation in the data, they are most appropriate for showing individual values (e.g. count data) rather than aggregates of several values (e.g. arithmetic means). Although variation can be shown through error bars, this is only appropriate if the data are normally distributed.
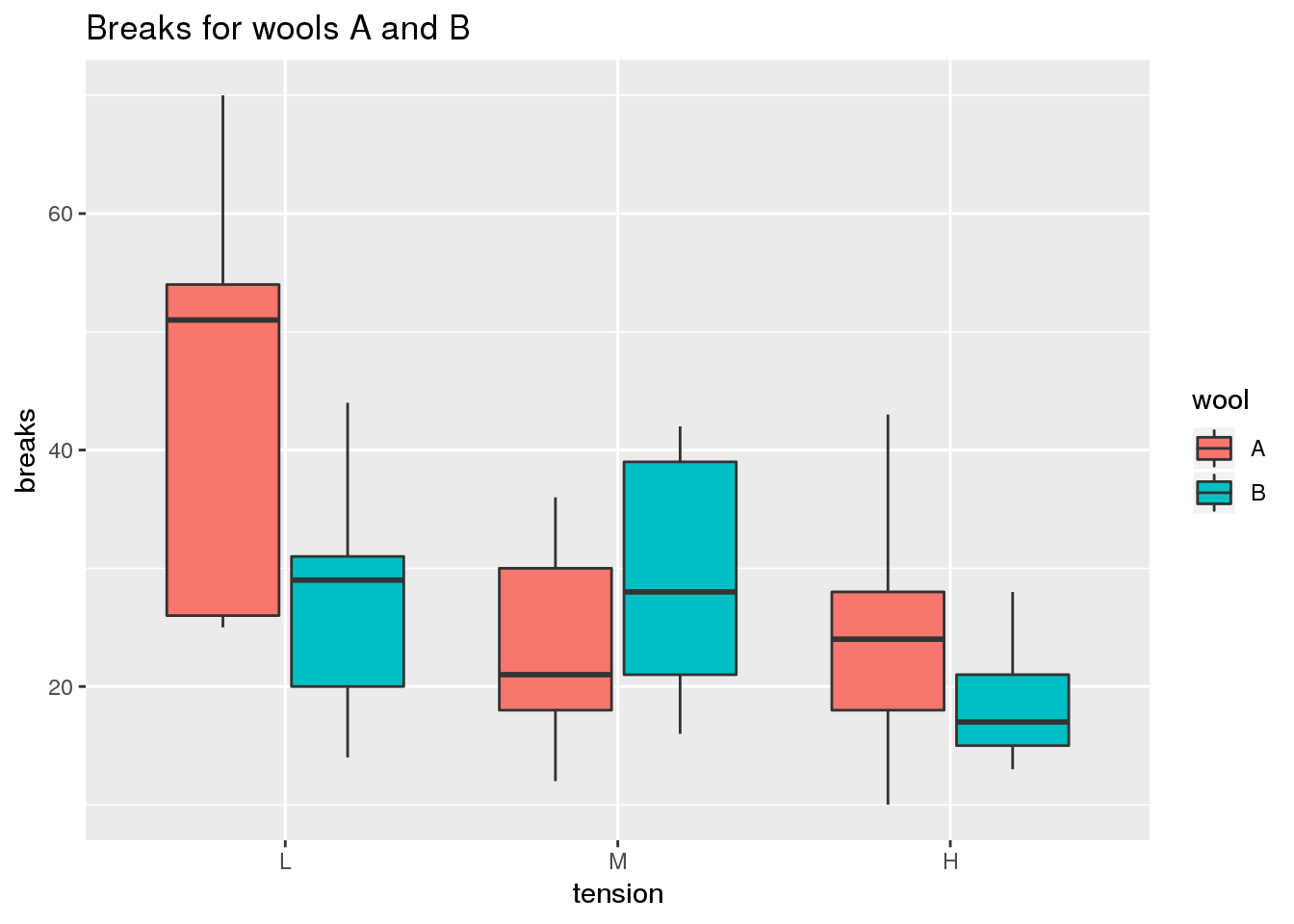
Comparing Medians and Inter-Quartile Ranges Using the Box Plot
The box plot is useful for comparing the quartiles of quantitative variables. More specifically, lower and upper ends of a box (the hinges) are defined by the first (Q1) and third quartile (Q3). The median (Q2) is shown as a horizontal line within the box. Additionally, outliers are indicated by the whiskers of the boxes whose definition is implementation-dependent. For example, in geom_boxplot
of ggplot2, whiskers are defined by the inter-quartile range (IQR = Q3 - Q1), extending no further than 1.5 * IQR.
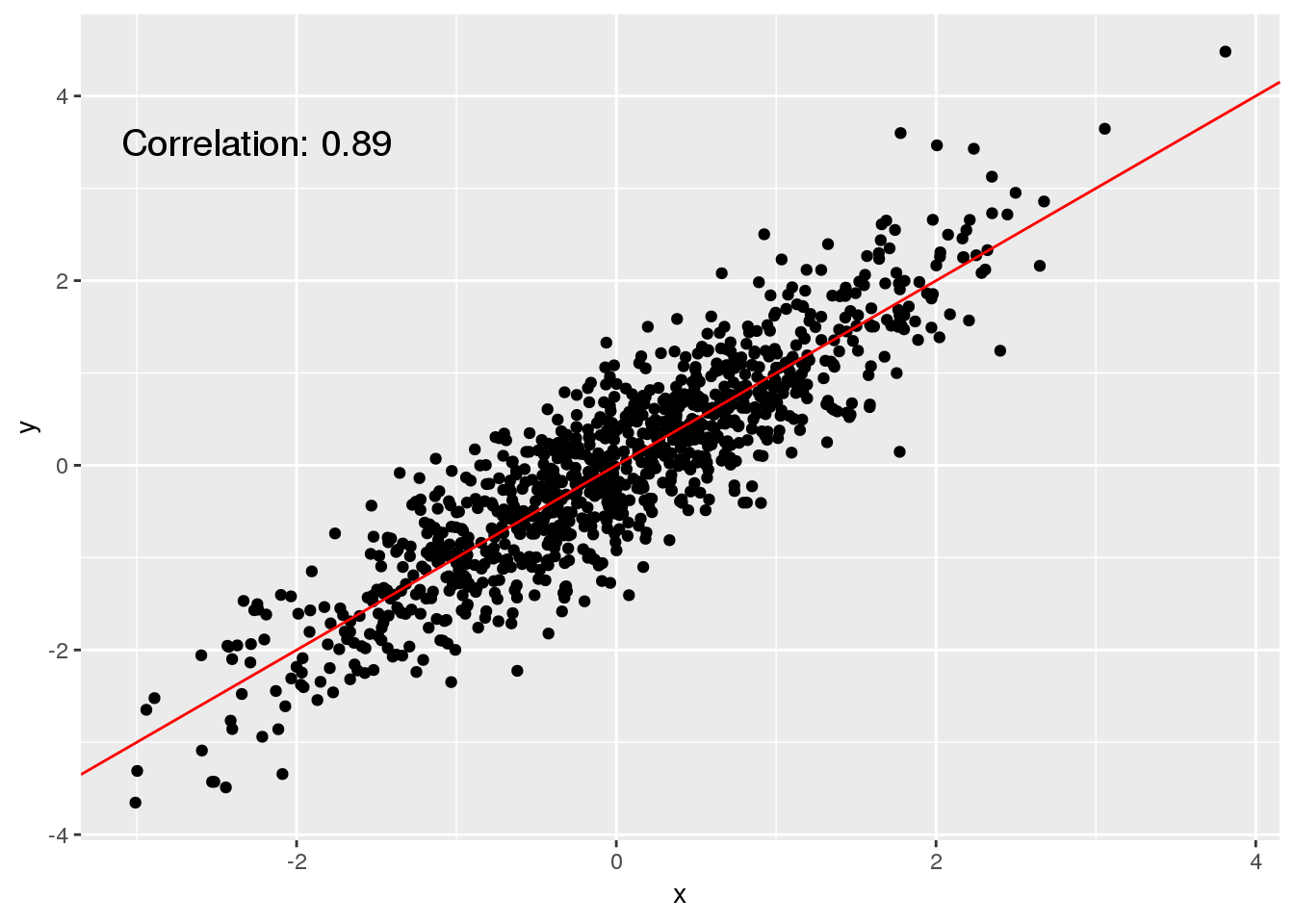
Visualizing Individual Data Points Using Scatter Plots
The scatter plot is probably the most simple type of plot that is available because it doesn’t do anything more than to show individual measurements as points in a plot. The scatter plot is particularly useful for investigating whether two variables are associated.
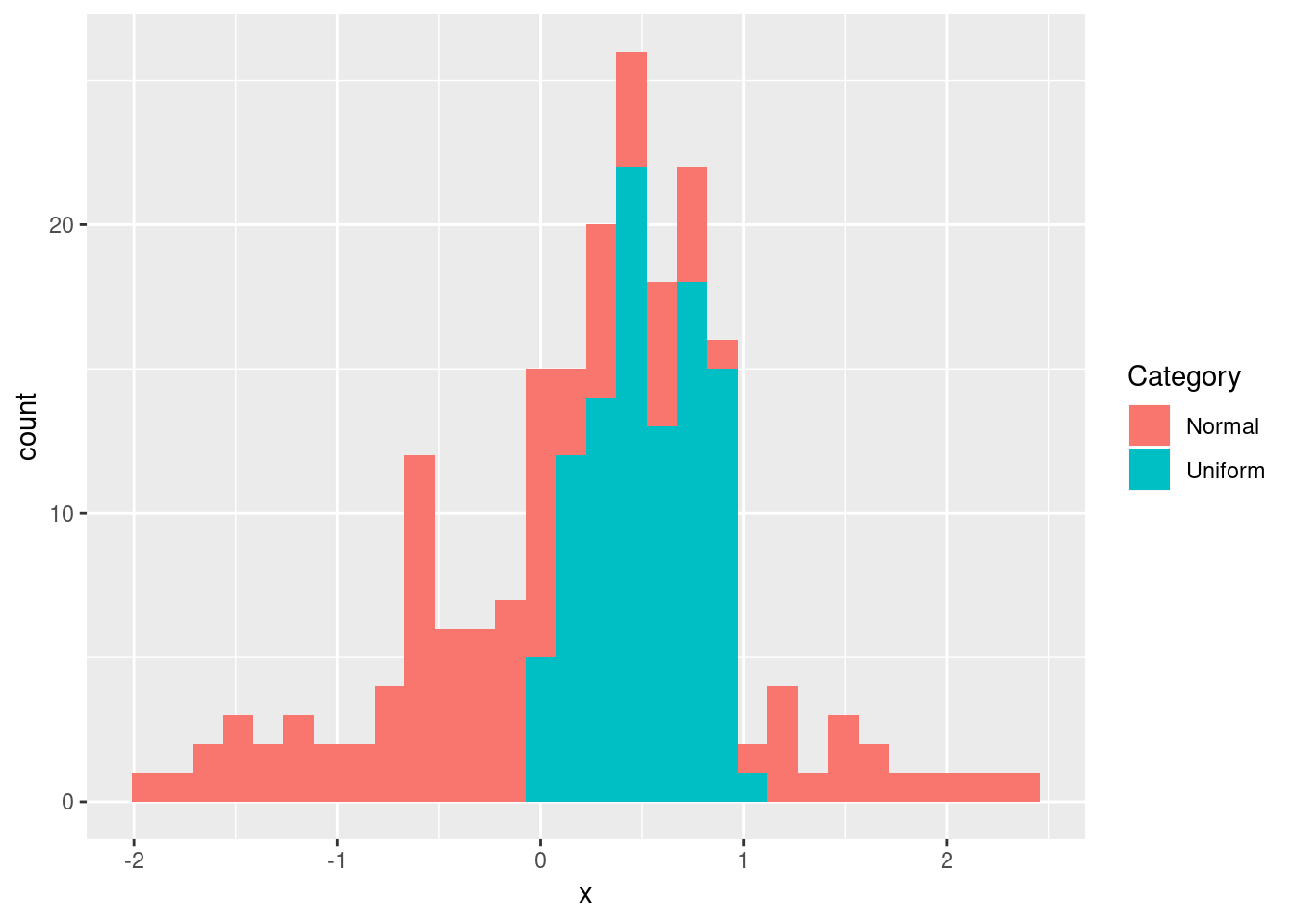
Determining the Distribution of Data Using Histograms
It is always useful to spend some time exploring a new data set before processing it further and analyzing it. One of the most convenient ways to get a feel for the data is plotting a histogram. The histogram is a tool for visualizing the frequency of measurements in terms of a bar plot. Here we’ll take a closer look at how the histogram can be used in R.